Science
MIT’s AI Decodes Proteins – Machine Learning Model News
Discover how MIT’s advanced machine learning model is revolutionizing the way scientists determine protein structures. Stay updated with MIT News.
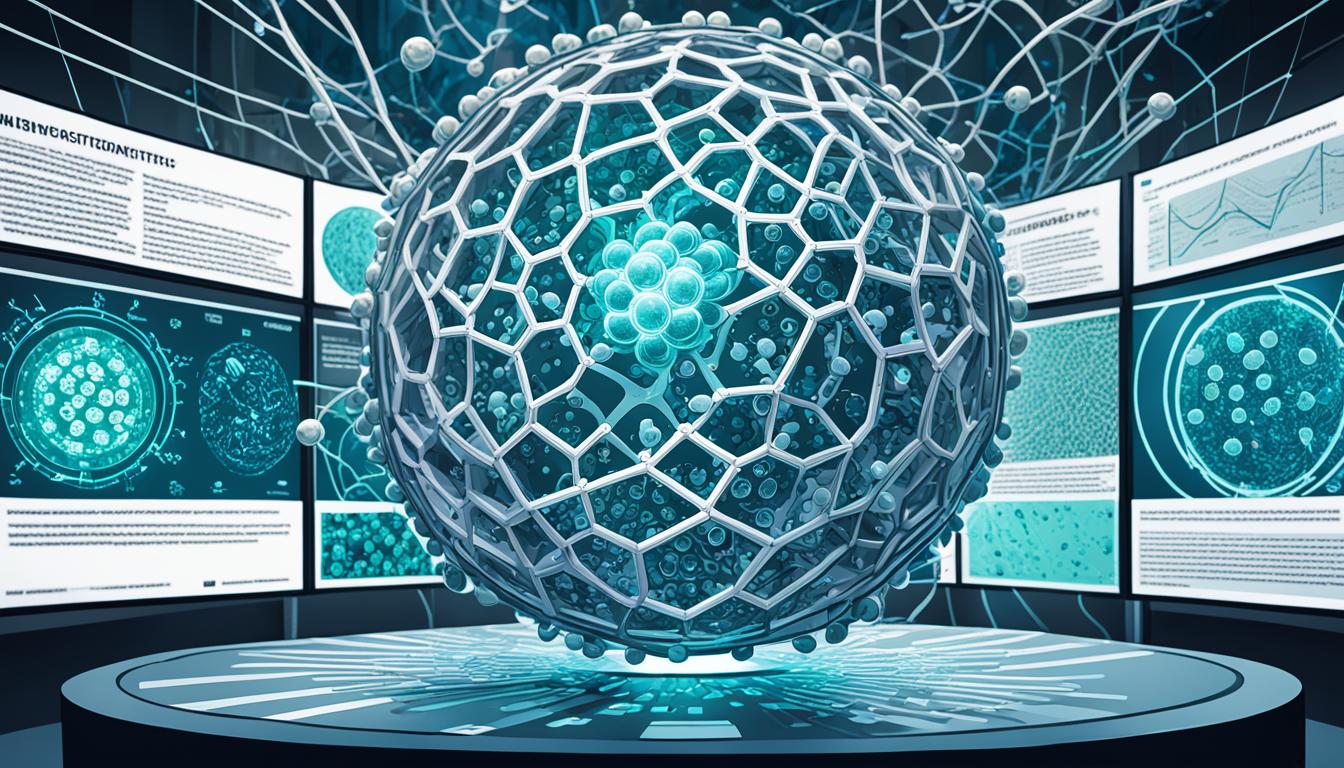
Imagine a world where the secrets of protein structures are easily revealed. A place where drug development is precise and within reach. MIT’s advancements make this future possible now.
Finding effective treatments is tough due to complex protein structures. Before, researchers spent lots of money and time on experiments to understand these proteins.
At MIT, a group led by Gabriele Corso and Hannes Stärk uses AI to decode proteins efficiently. Their machine learning model changes the game in drug development.
They created a tool that accurately predicts protein positions, far better than old methods. This tool’s success rate is impressive, beating traditional models by a large margin1.
This technology allows much of drug discovery to happen on computers1. It speeds up the process considerably. Now, researchers can find drug targets in a day instead of years.
What makes this AI unique? Corso and Stärk’s model can guess many outcomes, not just one1. This flexibility means it can handle uncertainty well, making better predictions.
Mit’s AI model is a big leap in understanding drugs and proteins. It opens new paths for creating medicines and exploring how cells work.
Scientists can now explore how drugs work with proteins more deeply. This leads to safer, more effective medicines. It marks progress in medical science and could help millions.
Key Takeaways:
- MIT researchers have developed an advanced machine learning model that uses AI to decode protein structures.
- DiffDock’s “blind docking” approach surpasses traditional models in accuracy, reaching 22% precision within 2 angstroms.
- The use of DiffDock in the drug discovery process saves significant time and costs, allowing for virtual drug target identification and mechanism of action characterization in just a day.
- DiffDock’s generative modeling approach enables multiple predictions, avoiding the limitation of searching for a single right answer and improving outcomes in the face of uncertainty1.
Understanding the Importance of Condensates in Drug Concentration
Condensates are special cell areas without membranes that form from tangled molecules. They turn into droplets inside cells, creating spaces for important molecular gatherings and functions2. Studies show that drugs can pile up in these condensates. This affects how well the drugs work3. By studying these condensates, scientists can pinpoint where in the cell a drug will go. This helps them make better drugs2. Knowing all about this is crucial for crafting drugs that are safe and hit their target precisely, especially in the condensates3.
The amount of drug in condensates depends on how molecules inside these spaces interact. The drug’s physical traits and what the condensate is made of play roles too2. Knowing about these interactions is key to understanding how drugs work inside cells. By making drugs that gather in the right condensates, scientists can make treatments more effective and reduce unwanted side effects3.
In one MIT study, researchers looked at where FDA-approved drugs ended up inside cells. They found these drugs don’t always go where expected3. For drugs to work well and be safe, they should gather where their target protein is3. This research stresses the need to think about where drugs collect in cells when designing them for the best results3.
Teams of AI experts and biotech firms, like Dewpoint, are working together. They use artificial intelligence to better understand how cells work and what goes wrong3. This team effort brings together knowledge from biology, chemistry, physics, and AI. They aim to get new insights into how drug concentration in cells can be improved3. This could lead to more effective treatments for many diseases3.
The study of condensates in drug concentration is giving new directions for drug creation and how to make them target cells more accurately. By looking closer at how drugs interact within condensates, scientists hope to enhance the effectiveness of drugs. This could lead to better treatments and safer medications for patients.
Predicting Drug Concentration in Condensates Using Machine Learning
MIT researchers are using machine learning to find drug concentrations in cellular condensates. This helps make drugs more effective and reduces side effects. They’re combining AI’s power with targeted treatments for better results.
They looked at how drugs pile up in cell compartments. The team noticed that drugs tend to gather in certain areas4.This discovery led them to study condensates’ role in drug localization.
The team tested over 1500 molecules across three condensate types: transcriptional, gene repression, and nucleolus4. Using machine learning, they figured out patterns that predict where drugs concentrate. This method could change how we predict drug locations based on their chemical structures.
Their machine learning model did well in predicting condensate drug levels. It even had some success with live cells4. This shows how complex the cellular world is, with many proteins changing the chemical scene.
They found that transcriptional condensates attract molecules with electron-rich rings4. This helps in designing better drugs and targeting them more effectively.
The Significance of Cellular Organization and Drug Concentration
This research shows how key it is to understand cell organization and drug concentration. Knowing about the proteins in cells can improve drug prediction models4. This could help make better drugs with fewer side effects.
It also shows the vital role of condensates in how drugs work and are developed. Understanding condensates’ chemistry is crucial for biology and finding new drugs4.
Implications for Drug Developers and Cellular Processes
The MIT team’s work could really help those making drugs and studying cell processes. Knowing how to predict drug levels in cells means better drug designs. This can make treatments more effective and safer4.
Last, understanding proteins’ physical and chemical traits can lead to better therapies. Controlling drug levels in cells could improve patient outcomes and cut down on side effects4.
AI Designing Novel Proteins with Specific Structural Features
MIT experts are pushing the boundaries of protein design using AI, focusing on creating proteins with unique structures5. They use advanced machine learning to predict amino acid sequences that achieve specific goals. Their work could lead to new materials that are not only effective but also environmentally friendly.
This approach in protein design is highly productive. In a few days, millions of new proteins can be made5. These creations closely match up to 60 percent of existing proteins5.
Even with ambitious goals, the AI models reliably produce realistic solutions5.
The team developed two kinds of models for detailed protein design5. This gives them precise control over the protein’s structure.
They also linked these models to an algorithm that predicts how a protein folds5. This connection helps in checking if the protein meets design criteria.
This pioneering work is supported by top organizations like the MIT-IBM Watson AI Lab and the U.S. Department of Energy5.
Advantages of AI Protein Design | References |
---|---|
1. Rapid generation of millions of novel proteins with specific structural features | 5 |
2. High overlap between newly designed and existing amino acid sequences, indicating synthesizability | 5 |
3. Production of realistic and probable solutions for physically impossible design targets | 5 |
4. Fine-grained control in protein design at both overall structural and amino acid levels | 5 |
5. Integration with protein folding algorithm for property determination and optimization | 5 |
Smoothing the Protein Fitness Landscape for Optimization
To make protein fitness better, MIT scientists suggest using graph methods to smooth the landscape. This makes finding better proteins easier and quicker. It helps find new proteins with enhanced fitness for certain traits.
They created a method called Gibbs sampling with Graph-based Smoothing (GGS). It smooths the fitness landscape and uses Gibbs sampling for top-notch outcomes6. This could change how we engineer proteins.
Imagine the protein landscape as complex ground with high and low spots. High points mean good fitness and low points mean poor. Traditional methods struggle because the landscape is rough and noisy. But, smoothing it removes small bumps, making optimizing easier.
GGS uses graph smoothing to make the landscape less noisy. It looks at nearby points to help find the best protein sequences6. This makes it easier to spot sequences with better fitness.
By adding Gibbs sampling, GGS explores many possible sequences at once. This broad search helps find really good sequences that other methods might miss6.
Using GGS has given better results in making proteins better. It has beaten earlier methods and improved the fitness for various protein features6. This could help in many areas, like medicine, bioengineering, and making new biological systems.
GGS shows how using machine learning and optimization can improve protein engineering. With computers and statistics, scientists can better understand the complex protein fitness landscape. This speeds up making new proteins with the traits we want.
Advancing Protein Optimization with Graph-Based Smoothing
Protein optimization is vital in fields like biotech and pharma. Scientists at MIT developed a method called Graph-based Smoothing (GGS)7. This method blends Gibbs sampling with graph signal processing to smooth the fitness landscape. It makes protein optimization algorithms work better.
Using GGS led to a 2.5 times improvement in fitness for the studied proteins7. It boosted performance in GFP benchmarks, raising fitness from 18% to 39%. Likewise, in AAV benchmarks, fitness jumped from 4% to 44%7. These gains show GGS as a top-notch method, outdoing other techniques in both GFP and AAV.
GGS does well because it uses a smooth energy function7. But, it’s not perfect for all optimization issues. Still, when combined with other methods like GWG, GGS gives excellent results in many situations7.
Turning fitness data into a form optimization algorithms can use is critical. Regularization helps sort out noisy fitness landscapes. This makes sure the algorithms work right7.
GGS’s use in protein optimization is a big step forward. It helps find high-quality proteins. Combining Gibbs sampling with graph-based smoothing opens up new ways to design and improve proteins7.
Graph-Based Smoothing in Protein Optimization
Graph-based smoothing has changed protein optimization. MIT researchers used it to estimate fitness landscapes from initial data8. The model they built can guess the rest of the landscape, making it easier to optimize proteins8.
They trained the model with data from about 1,000 green fluorescent protein (GFP) variants8. A CNN model helped predict optimized GFP sequences. These turned out to be 2.5 times fitter than the originals8.
This smoothing method also helped find new sequences for AAV viral capsids. Such advancements support industries like biotech and pharma in engineering proteins with specific roles8.
Advancements in Protein Optimization Models
Recent models like Chroma, RF Diffusion, and ESM-2 showcase progress in protein design. The RF Diffusion model, from the Baker Lab and MIT, created 1,000 protein designs9. ESM-2, by Meta AI FAIR, sets new records and helps with protein forms without needing Multiple Sequence Alignments9. Liu et al.’s MoleculeSTM even lets users edit molecular graphs based on descriptions9.
Such models can generate protein sequences and structures at the same time. ProtSEED, by Shi et al., works well in various design tasks9. Inverse folding models are also evolving, helping create sequences from structures9.
Machine learning is enhancing molecular dynamics and discovery too. GNNs have made a big difference in efficiency and accuracy. In addition, ML models like GraphCast by DeepMind and Pangu-Weather by Huawei are improving weather forecasting, beating traditional methods with quicker and more accurate predictions9.
Conclusion
MIT’s research is changing the pharmaceutical world. Their work in protein engineering is leading to new drugs and materials. They’re using machine learning to change how we see proteins. This could make drugs better and cheaper while bringing new therapies.
10Thanks to cryo-electron microscopy, scientists can now see proteins like never before. This shows how proteins look in 3D. MIT’s algorithms use this data to predict various protein structures. This is key in protein science. Their AI software can find all possible structures quickly, making protein analysis faster.
MIT’s work on ribosome assembly has shown how effective their methods are. They discovered a new ribosomal state. This could lead to new drugs and teaches us about cell functions.
This research doesn’t just impact protein engineering. It’s also making drug development faster and more affordable. Techniques like DiffDock are making it easier to find drug targets. Funding from the National Science Foundation, the National Institutes of Health, and others shows the value of MIT’s work11.
FAQ
What is the significance of condensates in drug concentration?
How does machine learning predict drug concentration in condensates?
How does AI design proteins with specific structural features?
What is graph-based smoothing in protein optimization?
How does graph-based smoothing improve protein optimization?

Science
Increased UFO Activity Over Popocatepetl Volcano
Discover why the mysterious increased-ufo-activity-over-popocatepetl-volcano is captivating UFO enthusiasts and skeptics alike.
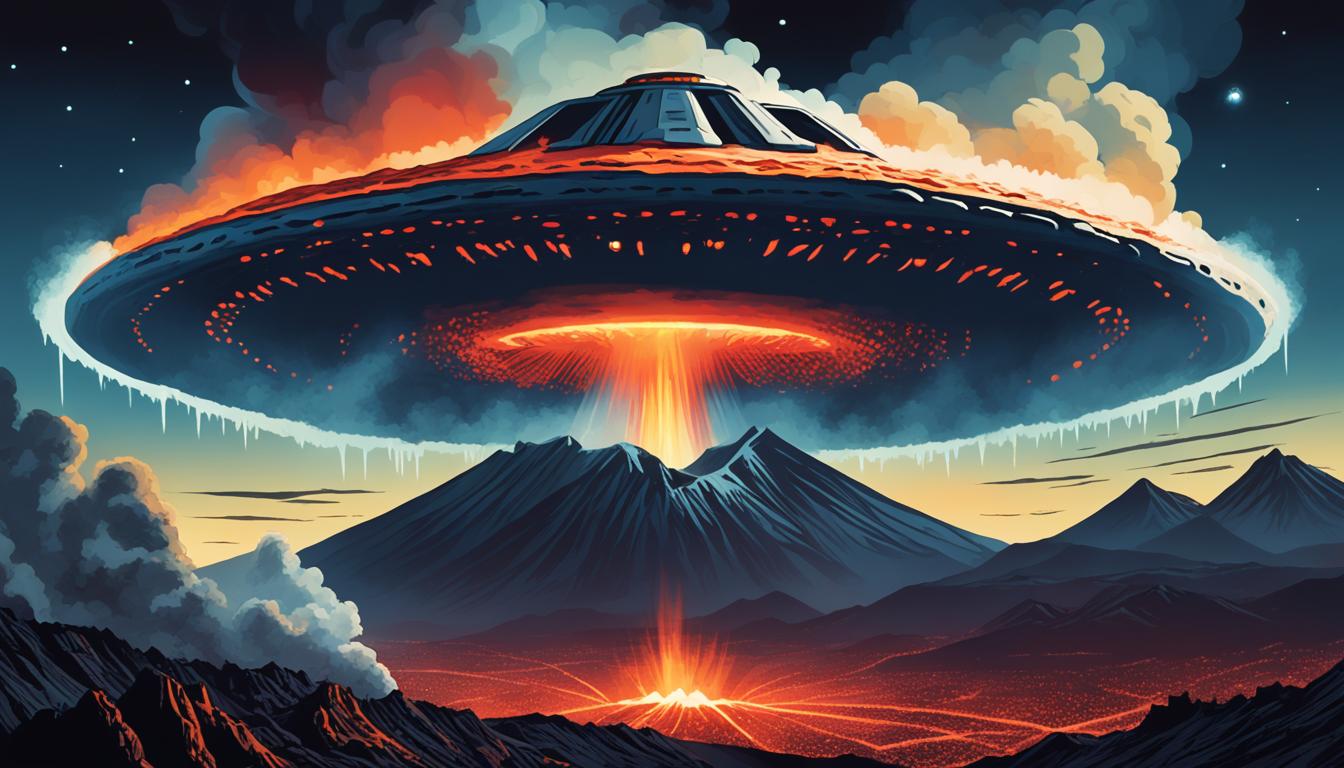
People all over the world are fascinated by UFO sightings. From strange lights to unexplained flying objects, these mysteries make us wonder. Recently, researchers found something interesting. There’s more UFO activity around active volcanoes in Latin America, especially around Mexico’s Popocatepetl volcano.
Locals call it “Popo.” It’s an active volcano close to Puebla, 45 miles southwest of Mexico City. It’s known for its eruptions and beauty. But lately, more UFO sightings near the volcano have caught everyone’s eye, from UFO fans to skeptics.
This rise in UFO sightings around Popocatepetl has been happening for years. In 1986, mountain climbers went into the volcano’s crater. They noted the crater’s rim is 5,250 meters high. Inside, they found a smaller crater and a warm lake on the crater’s floor, with a temperature of 29°C1.
More UFO evidence came to light later. In 1989, people visiting the volcano saw new fumaroles releasing steam and gas. These openings were on the main crater and its south flank. Steam shot up 20 meters high, smelling slightly of sulfur1.
In 1990, a seismic station was set up near Tlamacas, close to the volcano. It picked up small earthquakes around the volcano. These quakes showed that sulfur was condensing inside the crater1.
The UFO sightings kept increasing. By 1993, more steam was coming out of the crater. Researchers also noted the seismic station at Tlamacas showed normal seismic activity1.
In 1993, the people of Puebla noticed more steam plumes. The lake’s temperature inside the crater went from 29°C in 1986 to 51°C in 1993. Also, about 30 new vents were found, adding to the mystery of the volcano’s link to UFOs1.
In June 1993, the excitement grew. Climbers reached the summit on January 3. They saw bigger emissions from the volcano. The crater’s major and minor axes and the elevation difference were all measured, showing the volcano’s grand scale1.
Key Takeaways:
- The Popocatepetl volcano in Mexico has seen an increase in UFO activity, intriguing both UFO enthusiasts and skeptics.
- In 1986, mountain climbers discovered a warm lake on the E floor of the summit crater and measured the dimensions of the crater.
- Observations in 1989 revealed fumaroles emitting high-pressure gas and steam, while seismic activity persisted in 1990.
- In 1993, researchers noticed increased fumarolic activity and rising lake temperatures, accompanied by an uptick in plumes and larger emissions.
The Popocatepetl Volcano
Located in Mexico, Popocatepetl is a stratovolcano active since 19942. It stands tall at 17,797 feet, making it a key figure among the world’s volcanoes. Its location near Mexico City puts over 22 million people at risk.
The volcano is known for its toxic fumes, ash, and incandescent rocks2. It has disrupted life for nearly 30 years. Eruptions often force schools and airports to close. This includes Mexico City’s Benito Juarez and the Felipe Angeles airports3.
Due to regular eruptions, the alert level has been raised to “yellow phase three”3. This means we need better emergency plans as activity increases. A volcanic ash plume has even gone higher than 30,000 feet, making the concern greater3.
About 90% of the world’s volcanoes, including Popocatepetl, lack constant monitoring3. But, efforts to watch them are improving. The VOLCAT system uses satellite data for quick eruption alerts3.
UFO Sightings and Volcanic Hotspots
There’s an interesting link between UFO sightings and volcanic hotspots, especially in Latin America. It seems that volcanic activity might draw in UFOs. This results in more UFO sightings around these areas. A key spot for such activity is Mexico’s Popocatepetl volcano.
The Popocatepetl volcano stands tall at 17,800 feet4. It’s among Mexico’s most active volcanoes4. Its constant activity and eruptions catch the eye of UFO fans. Recently, two UFOs were reported entering the volcano’s crater4.
Yet, Popocatepetl isn’t alone in this. Other Latin American volcanic areas report UFO sightings too. For instance, Colima witnessed 11 UFO sightings from 1999 to 20115. The Michoacan-Guanajuato region had four sightings between 1943 and 19525. These reports suggest a strong link between volcanoes and UFOs.
Skinwalker Ranch in Utah is another spot known for UFO sightings. It has become famous for such activity. The ranch even inspired a TV series on the History Channel in 2020 titled “The Secret of Skinwalker Ranch”4. This series delves into the ranch’s mysteries and its UFO connections.
When UFOs are seen entering the Popocatepetl volcano, it sparks debate. The volcano’s interior reaches about 1,832 degrees Fahrenheit4. Some think this indicates the UFOs aren’t from Earth. They argue that such objects must withstand incredible heat, suggesting a non-human origin.
This link between UFOs and volcanic hotspots in Latin America opens up intriguing questions. Are these sightings explained by science or something more mysterious? Exploring these events further is key to finding answers.
Increased UFO Activity Over Popocatepetl Volcano
The Popocatepetl volcano in Mexico is a hotspot for UFO sightings. Enthusiasts and skeptics are drawn to this place. Strange lights and objects have been seen around the volcano, creating lots of talk about what they could be. While some people think there’s a simple explanation, the sheer number of sightings raises questions.
On January 8, a picture showed a UFO near the erupting volcano. This photo, by Luis Guerra, became hugely popular online. The person who took it lives close to a UFO monument in Atlixco, Puebla, Mexico6.
The volcano, standing around 10,000 feet tall, adds mystery to these occurrences. Atlixco is known for spooky events, like UFOs and Chupacabra sightings7. In the ’90s, this place was a major UFO spot. A special UFO sculpture stands on a hill known for sightings since 20017.
These recent UFO reports near the Popocatepetl are not alone. Mexico’s history is rich with UFO stories, from Tula to sightings across Mexico and the U.S. These reports keep people interested in the UFO phenomenon6.
UFO sightings aren’t just in Mexico. They’ve been reported worldwide for centuries. In the 1600s, Puritans recorded them without mentioning aliens. The famous 1947 Roswell event led to a boom in sighting reports8. Places like Wycliffe Well, Warminster, and the Nazca Lines also witness these mysterious events, keeping our interest in possible extraterrestrial life alive8.
Location | Statistical Data |
---|---|
Popocatepetl Volcano, Mexico | Increased UFO activity during eruptions |
Atlixco, Mexico | Known as a paranormal hotspot with numerous UFO sightings |
Tula, Hidalgo | Prior reported flying saucer sighting in Mexico |
Mexico and U.S. | Reports of flicker in orange lights in various cities |
People’s growing interest in UFOs is clear from the viral photos and stories. Despite doubts, the activity near Popocatepetl and elsewhere keeps many hooked. As we learn more about space, our hunt for answers about these unidentified objects continues to excite us.
Skepticism and Explanations
Many skeptics attribute UFO sightings to common, earthly sources. For example, they suggest the UFOs seen over Popocatepetl volcano are just airplanes. But, the strong evidence and stories from witnesses call for a deeper look.
A lot of people have seen UFOs near Popocatepetl. This has happened over several years9. These experiences are hard to ignore. Researchers from Harvard found unusual seismic shifts and electromagnetic fields in the volcano9. It hints at an energy source that’s not from natural volcanic actions, maybe even man-made9. These unusual signs make us question the usual explanations and consider new ideas.
There’s a theory that aliens might be using Popocatepetl as a base, which has made many scientists skeptical9. They think the UFOs might just be mistaken identities or optical illusions. Yet, it’s key to stay open and check these claims carefully and without bias.
Challenging Common Misconceptions
The Harvard group showed how bringing different scientists together is valuable9. They combine their knowledge to figure out the phenomena’s real cause. This kind of science can help answer doubts with facts and real evidence.
Encouraging Further Exploration
Looking forward, new tech like deep radar and satellites might shed light on the UFOs near Popocatepetl9. Using these tools, we can learn more about the mysterious sights. Plus, teamwork across countries and from many labs will help keep the study honest and open9. Working together, we can get to the bottom of this intriguing mystery.
Common Explanations | Counter Arguments |
---|---|
UFO sightings are misidentifications or illusions caused by natural phenomena. | Eyewitness testimonies and advanced monitoring equipment provide compelling evidence of anomalous activity. |
The flying object captured in photos and videos is an airplane. | The research conducted by the Harvard team suggests an additional source of energy beyond typical volcanic activity9. |
There is no concrete proof of extraterrestrial presence or advanced civilizations. | The anomalous seismic readings and electromagnetic pulses point to potential artificial origins9. |
As investigations go on, it’s crucial to weigh skepticism against the facts and stories we have. While there may be normal reasons for these events, the oddities at Popocatepetl deserve more study and scientific work.
Underground Civilizations?
A rare idea about UFOs near the Popocatepetl volcano is the cryptoterrestrial hypothesis. It suggests there might be smart beings living underground. These beings could be behind the UFO sightings. Scientists doubt this theory, but it’s worth thinking about.
Those who believe in this theory say these underground societies are ancient. They might have secret bases in big volcanoes like Popocatepetl. They use advanced tech to stay hidden and explore Earth.
The Hotspot of Popocatepetl Volcano
The Popocatepetl volcano in Mexico is famous for UFO sightings. There’s a long history of mysterious flying object reports here. UFO fans and experts find it very intriguing.
Statistics10 show that since 1969, there have been over 200,000 UFO reports. The US reports the most. The Department of Defense’s AARO office looks into these cases. Over 800 involve flight safety issues.
This unusual hypothesis gives a different reason for the UFOs around Popocatepetl. Maybe underground communities use special tech for this. Most scientists don’t agree, though.
Connecting the Dots
The idea of hidden civilizations beneath us is intriguing. We know so little about Earth’s history. Missing fossil records make us wonder what’s hidden below.
Supporters of the cryptoterrestrial hypothesis11 point to mysterious archaeological finds. Like a Stonehenge-like structure in Lake Michigan and the Ganonpadon site, which could be very old. These may hint at a secret past.
Statistical data11 links half of UFO sightings to water. The Disclosure Act even talks about submariners seeing UFOs. This supports the theory of active underwater and underground beings.
We can’t prove if underground societies exist. Yet, it’s valuable to stay open to new explanations for UFOs near Popocatepetl. Exploring these ideas can unlock more mysteries of our planet.
Other Theories Surrounding UFOs
Besides the cryptoterrestrial idea, other thoughts exist about UFOs and where they may come from. One theory is the interdimensional hypothesis. It thinks UFOs could be from dimensions outside what we normally see. According to this, such beings can move between dimensions and visit us, seen as UFOs. This idea suggests they have technology to travel through dimensions. This would explain how they suddenly show up and vanish10.
Then, there’s the ultraterrestrial hypothesis, which is broader. It says that non-human intelligence might already be on Earth, perhaps underground or near us, like the moon. Some believe these beings are from old civilizations or totally different life forms from us. They say these beings stay hidden in hard-to-reach places. That’s why we don’t know much about them10.
These theories change how we see extraterrestrial life and give new reasons for UFO sightings. While one idea talks about beings from other dimensions, the other believes in non-human intelligence among us. Both suggest exciting ways to view UFOs10.
Safety Concerns and Impact on Daily Life
Increased UFO sightings near the Popocatepetl volcano have caused worry. These sightings and eruptions affect those living nearby greatly. Ash from the volcano threatens people’s health.
Breathing in volcanic ash can harm your lungs, especially if you already have breathing issues. It can make people cough and wheeze, making it hard to breathe. Nearby residents have complained about these health problems due to the ash1.
This ash also lands on buildings, cars, and more. It can break engines and machines if not cleaned off. People have to wear masks and clean their belongings to deal with the ash1.
People living close to the volcano need to change how they live. They’re told to stay inside when the volcano erupts and keep windows shut. They also need to stop outdoor activities to stay safe from ash and health issues1.
The authorities keep an eye on the volcano to keep people safe. They tell people what to do through education and communication. This helps everyone know how to stay safe from the volcano’s ash1.
Volcanic Ash Safety Guidelines
Precautions | Actions |
---|---|
Stay indoors | Avoid outdoor activities during eruptions to minimize ash exposure |
Close windows and doors | Prevent the entry of ash into living spaces |
Wear masks | Use masks approved for filtering fine and small particles during ashfall |
Clean surfaces | Regularly remove ash from roofs, vehicles, and outdoor furniture |
Follow official updates | Stay informed about the volcano’s activity through reliable sources |
It’s very important for people to listen to the guidelines from authorities. Taking these steps can keep them safe during high volcanic activity. By being well-informed and ready, they can protect their health from volcanic ash1.
Research and Investigation
The Popocatepetl volcano has seen a rise in UFO activity, catching the eye of many. Scientists, UFO buffs, and skeptics are all drawn in. They’re working together to figure out what these UFOs might be and how they relate to the volcano.
Studying these mysteries involves a lot of different steps. Researchers hit the fields, listen to those who’ve seen UFOs, and look at photos and videos. They also check data from equipment that watches over earthquakes and volcanoes, seeing if it matches up with UFO reports.
They’re diving deep into what these UFOs do, where they might come from, and how they act around volcanoes. Could they be aliens, something from another dimension, or just advanced tech we don’t know about yet? That’s what they’re trying to find out.
- They’re looking into weird magnetic and physical changes that happen when UFOs show up near volcanoes12. This could tell us how UFOs move.
- They also study UFO reports from the past, especially around volcanoes all over the world, and compare them to what’s happening at Popocatepetl12. This might show patterns that explain these mysteries.
- This work isn’t done alone. It includes help from experts in spooky stuff and space life from around the globe12. Sharing knowledge helps everyone get closer to the truth.
Even though some still doubt, thinking UFOs are just mistakes or natural things, the evidence is too strong to ignore. As we get better at studying this stuff, the secrets of UFOs near the Popocatepetl volcano might finally be revealed.
Research Findings | Reference |
---|---|
The electromagnetic anomalies detected during UFO sightings near volcanoes provide clues about the propulsion systems of these unidentified objects. | 12 |
Comparative analysis of historical UFO sightings in volcanic regions worldwide contributes to understanding the nature and purpose of the observed UFOs near Popocatepetl. | 12 |
Collaboration with international research organizations specialized in extraterrestrial studies enriches the investigation into UFO sightings near volcanoes. | 12 |
Global Interest and Media Attention
UFO sightings have sparked interest worldwide and drawn a lot of media focus. Across the globe, people have reported seeing strange things in the sky. This has reignited our curiosity and interest in otherworldly phenomena.
Thanks to the internet and social media, news about these sightings spreads fast. People everywhere can share their UFO stories, photos, and videos. The web has become a bustling place for talks, debates, and guesses about these strange sightings.
During the 2020 Covid-19 pandemic, UFO sightings seemed to increase. More people were outside, looking up at the sky, because of lockdowns13. This spike in sightings during such a unique time got more people and the media interested in UFOs.
Traditional and digital news have covered these events in detail. They show eyewitness stories, photos, and videos as proof. Social media, in particular, helps spread these stories wide, making some UFO sightings famous.
A video from a total solar eclipse in Texas caught everyone’s eye and went viral on social media14. Incidents like this, with video proof, make people want to learn more about the possibility of life in space.
People all over the world have seen strange lights that move in unique patterns. These stories add to the mystery and interest in UFOs14. When people share their experiences and show pictures, it makes others wonder and guess about what they’re seeing.
The media talks a lot about UFOs too. Figures like Tucker Carlson discuss bold ideas about UFOs on their shows. This kind of attention makes even more people curious and interested in the topic14.
Projects like SETI scan the sky for signs of life beyond Earth, making news worldwide. The involvement of scientists in researching these mysteries lends credibility. It also sparks even more interest in the possibility of meeting beings from other planets14.
The fascination with UFOs grows as more people talk about and explore these mysteries. Media plays a big part in keeping the excitement alive. Thus, more folks become part of a community that’s keen on learning about life beyond Earth. This global curiosity ensures that interest in UFO sightings will only get stronger over time.
Conclusion
UFO sightings over the Popocatepetl volcano have caught both fans and skeptics’ eyes. Data from different places151617 show more sightings between 2018 and 2019. Then, sightings dropped in 2018 but went up again in 2019. This pattern raises questions and thoughts.
Some people try to explain these sightings in simple ways. Yet, the sightings happen too often and too regularly to be just brushed off. The documentary “Volanic UFO Mysteries,” with journalist Jaime Maussan15, talks to experts and those who saw the UFOs themselves. It dives deep into the mystery.
People have many ideas, like alien doorways or strange magnetic effects17. These theories keep the intrigue around the volcano alive. As more research is done, the strange UFOs at the Popocatepetl volcano get more and more interesting1517.
FAQ
Are there any scientific explanations for the increased UFO activity over the Popocatepetl volcano?
Could the increased UFO activity be related to volcanic activity?
What are some theories that explain the increased UFO activity?
Are there any safety concerns for residents near the Popocatepetl volcano?
How are researchers studying the increased UFO activity?
Has the increased UFO activity over the Popocatepetl volcano received media attention?
Science
Russia and Space Collaboration: Global Impact
Discover how russia-and-space-collaboration shapes global aerospace dynamics and its far-reaching impacts on international relations and technology.
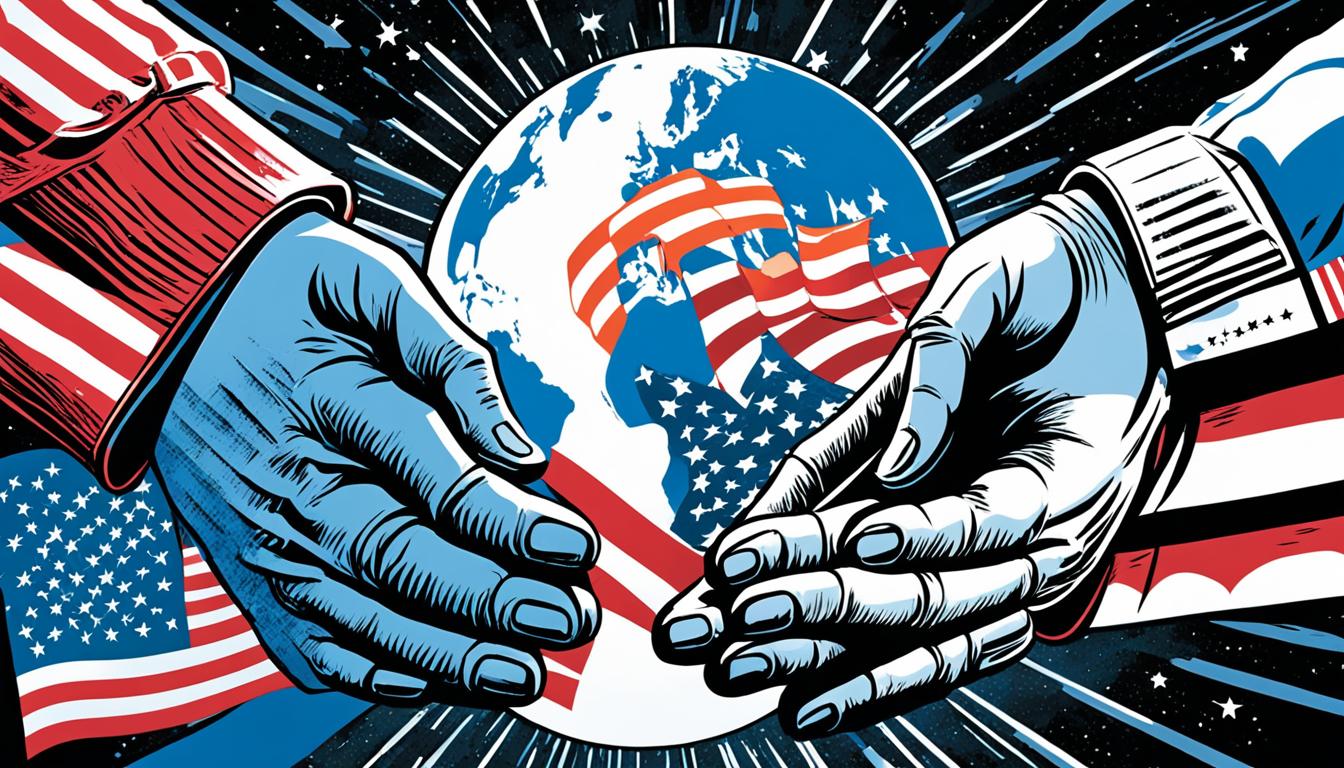
Russia has been a key player in space collaboration, impacting the world of aerospace, international ties, and technology. Let’s look at the various roles Russia has played in this field.
Think about a space station in orbit with astronauts from many nations working together. They do important research and explore new frontages. This places is the International Space Station (ISS), a beacon of joint effort and scientific progress1.
In the ISS program, Russia is a leading partner. It helps keep this incredible project running. Its teamwork with space agencies from the USA, Europe, Japan, and Canada breaks down global barriers1.
The ISS is not just for research but also shows how well countries can work together. Astronauts from 18 countries have visited it, showing how many nations are involved in this project1.
Russia’s role in the ISS and other partnerships has greatly affected aerospace technology. For example, a 2019 China-Russia deal gave China access to special missile defense tech. This deal shows how global space competition is changing and its effects on world relations2.
Also, Russia and China have teamed up on satellite and moon missions. In 2014, they agreed to work on satellite navigation, helping each other improve systems. And in 2021, they planned together for a moon research base2.
This closer Russia-China space bond signals a new phase, with China becoming more prominent. Such teamwork could challenge U.S. defenses against missiles, increasing the chance of global conflicts2.(`p`>
Looking deeper into Russia’s space collaboration, we’ll see its effects on aerospace industries, nonproliferation, U.S.-Russia relations, and international politics. It’s a complex mix of competition and teamwork in space21.
Key Takeaways:
- Russia’s space collaborations, especially through the ISS, greatly influence global aerospace and international politics1.
- The ISS combines flights, launch sites, operations, training, and science from all over the globe1.
- A total of 18 countries have sent astronauts to the ISS, showing widespread global involvement1.
- Space collaboration between Russia and China is evolving, with China leading, thanks to Russian support2.
- This teamwork could make it harder for the U.S. to control missile threats, potentially heightening global tensions2.
The Rationales for Expanded Space Cooperation
Russia and other countries, like the United States, are joining forces in space for many reasons. These include improving space tech, saving money, and enhancing foreign relations and security.
One main reason for this teamwork is to use Russia’s space knowledge to boost the U.S. space program. The U.S. benefits from Russia’s tech and infrastructure, which can lead to big achievements in space exploration. This partnership helps share ideas and solutions, cutting costs by doing missions together and using the same facilities3.
In 1993, Russia was asked to join the team building the international space station. This move, involving the U.S., Japan, Canada, and European countries, built a strong base for space teamwork. It helped improve relationships between countries and encouraged global cooperation in space3.
Security is another big reason behind this cooperation. Countries work together to keep space safe by sharing info and research. This teamwork protects space assets and promotes peace and stability up there3.
Money matters also drive countries to join forces in space. By sharing the costs and working together on research, they get more value for their money. This helps grow the economy by creating new tech, business deals, and industries related to space3.
There are many reasons why countries are working together more in space. They want to improve their space programs, save money, support foreign policy, boost security, and grow the economy. By combining their strengths, they can explore space further, learn more, and use outer space better for humanity’s progress.
Impacts on Russian Aerospace Industrial Base
Russia’s aerospace base has grown thanks to its work with other countries in space. These teams have shared technology and pushed aerospace skills higher. They have also helped in keeping space peaceful.
Russia and its partners, like China, have put a lot of money into space. In 2023, China spent $14.15 billion on its space projects, up 19% from the year before. Russia spent $3.41 billion, a bit less than in 20224. This shows both countries’ strong interest in discovering more about space.
Russia’s work with China and others has led to the sharing of advanced technology. This sharing means Russian aerospace can make new, top-notch technology. For example, Russia and China’s plan to build a nuclear power plant on the Moon by 20354. It shows how these countries work together to reach new heights in space.
Such cooperation in space has helped keep space peaceful. By working together, countries like Russia help make sure space is used in a good way. At places like the UN Security Council5, they talk about keeping space for peace and science.
But, Russia’s aerospace base does face problems. For instance, its satellite navigation system is getting old5. Many satellites are not working well because they’re outdated. This points to a need for more investment in space tech.
To end, working together in space has greatly helped Russia’s aerospace. It has allowed for sharing technology, improving skills, and promoting peace in space4. Despite some issues, Russia’s role in space shows its dedication to bettering aerospace and joining global space efforts.
Impacts on Nonproliferation and Technology Transfer
Space cooperation between Russia and other countries has made a big difference in stopping the spread of nuclear weapons and sharing technology6. By working together, they have spread knowledge and technology. This helps the world work towards preventing the spread of nuclear arms and improving technology.
The Nuclear Non-Proliferation Treaty was established in 1968 by nuclear and non-nuclear states. Its goal was to stop nuclear tech from spreading6. It aimed to keep nuclear weapons and technology from being passed around. The International Atomic Energy Agency (IAEA) checks to make sure nuclear tech doesn’t get spread wrongly6.
However, countries like France and China, along with others, didn’t join the NPT. This affected how well the treaty worked6.
Space collaboration has also boosted the sharing of technologies between nations. This exchange has improved areas such as aerospace technology. It strengthens global efforts to stop nuclear tech from being misused, by helping countries use nuclear tech safely and peacefully6.
International agreements like the 123 Agreement support nuclear cooperation for peaceful purposes7. The NPT, which started in 1970, remains vital for stopping the spread of nuclear weapons7.
Programs like the G-8 Global Partnership and UN Security Council Resolution 1540 focus on stopping weapons of mass destruction from spreading. They also help countries follow non-proliferation rules7. Conventions like the CPPNM and the International Convention on Nuclear Terrorism ensure nuclear materials are safe and fight against nuclear terrorism7.
The Nonproliferation Review is a key resource for those making policies and for scholars8. It publishes articles on international treaties like the NPT, focusing on important issues related to nuclear security and weapons spread8.
It also encourages discussions and research through book reviews related to stopping nuclear arms spread8. The Doreen and Jim McElvany Nonproliferation Prize rewards authors of standout submissions, highlighting the value of research in this area8.
In summary, space cooperation plays a crucial role in stopping nuclear weapons spread and in sharing technology. Through collaborations and efforts, countries share their knowledge and technologies. This aids in achieving global goals to prevent nuclear proliferation and enhances developments in many areas.
Impacts on Overall U.S.-Russian Relations
Space collaboration has brought the United States and Russia closer, creating a path for international teamwork. Working together in space has helped these two countries build strong relations. It has strengthened their ties, showing how teamwork can lead to positive outcomes.
The International Space Station (ISS) is a great example of U.S.-Russian cooperation. Right now, seven people live on the ISS. This crew includes four Americans, two Russians, and one German9. Their work together highlights the level of trust and international cooperation in space exploration.
Russia plays a vital role in keeping the ISS in orbit9. This shared duty shows the deep trust and reliance between the United States and Russia. It proves that their space collaboration goes beyond political issues, underlining its importance.
There have been hurdles, like Russia’s uncertainty about continuing the collaboration beyond 2024. The U.S., however, plans to support the ISS until 20309. Despite these challenges, joint ventures, such as commercial partnerships, help bridge gaps. Working with companies like SpaceX has lessened the U.S.’s dependence on Russia for space trips. This shows how flexible and strong their space relationship is9.
Political tensions and sanctions on Russia can affect space cooperation. For example, the European Space Agency’s Mars mission, needing a Russian rocket, faced doubts due to political issues9. Also, a planned Russian launch for the British company OneWeb got canceled. These situations show how politics can influence space collaboration9.
In conclusion, space collaboration between the U.S. and Russia has deeply influenced their relationship. Their shared space missions have fostered international diplomacy, showing how countries can work together above political differences. Yet, geopolitical tensions can challenge this teamwork. It’s essential to keep striving to maintain and enhance the U.S.-Russian bond in space.
The New Space Race and Global Geopolitics
The new space race is big in world politics and the way countries interact. Nations are pushing hard into space, making it a part of global politics. This shows how science, technology, and political power are all connected.
Countries like the US, China, and Russia are all in this space race. They all want to achieve their own goals. This competition is not just about exploring space. It also affects who has power in the world and how countries work together.
A big reason for the race is to achieve new tech and scientific discoveries. Over 80 countries are now in space, showing a big jump in global interest10. In the US, private businesses are spending more on space research. This shows that companies also play a big role in space exploration10.
Space mining is also getting attention, especially with China’s large metal reserves10. Mining in space could help countries depend less on Chinese resources.
The Moon could help meet the world’s energy needs with its helium-310. This could be a game-changer for how we get energy in the future.
Satellites are more important than ever for daily life and keeping countries safe. The UK could lose a lot of money if its satellites were ever disrupted10. This shows how crucial space technology is to our world.
While countries compete in space, working together has always helped humanity. The International Space Station is a great example of what can be achieved together10. Working together in space can help us all understand more, make new scientific discoveries, and promote peace.
The space race is changing how countries relate to each other and the balance of power. As nations try to be the best in space, working together becomes even more important. This competition is where science, tech, and politics meet. It will shape our future on Earth and in space.
Key Points | Statistical Data Reference |
---|---|
Over 80 countries currently have a presence in space | 10 |
Private companies in the USA have significantly increased their spending on research and development | 10 |
China holds a third of theoutgoing links world’s known reserves of certain metals | 10 |
The Moon is believed to contain vast reserves of helium-3 | 10 |
The UK stands to lose an estimated £1 billion per day if satellites are disrupted | 10 |
Collaboration in space exploration has historically led to breakthroughs benefiting humanity | 10 |
Tensions and Collaboration in Space
Space activities come with tension and cooperation as countries want to be leading and grow in science. But, lacking global rules often causes conflicts and competition. Yet, working together is key for space exploration and discovering new science.
The International Space Station (ISS) shows how well collaboration works in space. Right now, there are four US astronauts, three Chinese astronauts, and three Russian cosmonauts on the ISS. This diverse group11helps share knowledge and understanding across nations.
In 2022, Russia talked about leaving the ISS by 2024, later pushed to 202811. This move worries many about future teamwork and the science gained from working together.
The Role of Collaboration in Mitigating Tensions
Working together in space is not just for science but also helps reduce tension between countries. It’s very important to have clear, agreed-upon rules to prevent conflicts and keep peace.
Private space firms like SpaceX, led by Elon Musk and worth $125 to $140 billion, could help keep the ISS going if Russia leaves11. SpaceX might support the station with tools or money. It shows how important different partners and the private sector are for the future of space travel.
Evolution of Strategic Partnerships
The space industry’s changing nature leads to new partnerships. The European Space Agency (ESA) is thinking about using its own launchers instead of Russia’s “Soyuz” capsules11. This possibility marks a change in partnerships and strategies in space.
China is becoming a big player in space, challenging the usual leaders like the USA and Russia11. With its successful Moon missions and its own space station, China’s growth advocates for more global cooperation. This will help keep space exploration peaceful and fruitful.
Conclusion
Since 1957, Russia’s work with other countries in space has changed the world. The USSR launched the first satellite, leading to big achievements like the first person in space and the first Mars mission1213. These steps have pushed the boundaries of space technology and started a global competition to explore space.
Even with political tensions, working together in space is key for new discoveries and peaceful exploration. The United States and Russia, for example, teamed up for the Apollo-Soyuz mission in 1975. This mission marked the end of the space race in a symbolic way12. Nowadays, making rules and working internationally are crucial for the future in space.
Russia’s ongoing participation in space is important for the world’s approach to space. As China and Russia grow their space skills, working together becomes more critical. The U.S. Space Force sees the value in partnering with private companies. This helps make the most of new technologies and strengthens space security14. Combining commercial space technologies can lower costs, create jobs, and help many industries14. Also, the USSF’s work with allies, schools, and businesses shows a strong commitment to solving space problems14.
In the end, Russia’s work in space has deeply influenced aerospace, international relations, and technology worldwide. As countries keep pushing into space and growing their skills, working together will be essential. It ensures the ongoing growth of science and peaceful space exploration.
FAQ
What is the impact of Russia’s collaboration in space?
What are the rationales for expanded space cooperation?
How has Russia’s collaboration in space impacted its aerospace industrial base?
What are the impacts of space collaboration on nonproliferation and technology transfer?
How has space collaboration impacted overall U.S.-Russian relations?
How is the new space race shaping global geopolitics?
What are the tensions and importance of collaboration in space?
What is the conclusion regarding Russia and space collaboration’s global impact?